2024 was a year of immense growth for Bluesky. We launched the app publicly in February and gained over 23M users by the end of the year. With this growth came anticipated challenges in scaling Trust & Safety, from adding workstreams to adapting to new harms.
Throughout 2024, our Trust & Safety team has worked to protect our growing userbase and uphold our community standards. Our approach has focused on assessing potential harms based on both their frequency and severity, allowing us to direct our resources to where they can have the greatest impact. Looking ahead to 2025, we're investing in stronger proactive detection systems to complement user reporting, as a growing network needs multiple detection methods to rapidly identify and address harmful content. In Q1, we'll be sharing a draft of updated Guidelines as we continue adapting to our community’s needs.
Overview
In 2024, Bluesky grew from 2.89M users to 25.94M users. In addition to users hosted on Bluesky’s infrastructure, there are over 4,000 users running their own infrastructure (Personal Data Servers), self-hosting their content, posts, and data.
To meet the demands caused by user growth, we’ve increased our moderation team to roughly 100 moderators and continue to hire more staff. Some moderators specialize in particular policy areas, such as dedicated agents for child safety. Our moderation team is staffed 24/7 and reviews user reports around the clock. This is a tough job, as moderators are consistently exposed to graphic content. At the start of September 2024, we began providing psychological counselling to alleviate the burden of viewing this content.
Reports
In 2024, users submitted 6.48M reports to Bluesky’s moderation service. That’s a 17x increase from the previous year — in 2023, users submitted 358K reports total. The volume of user reports increased with user growth and was non-linear, as the graph of report volume below shows:
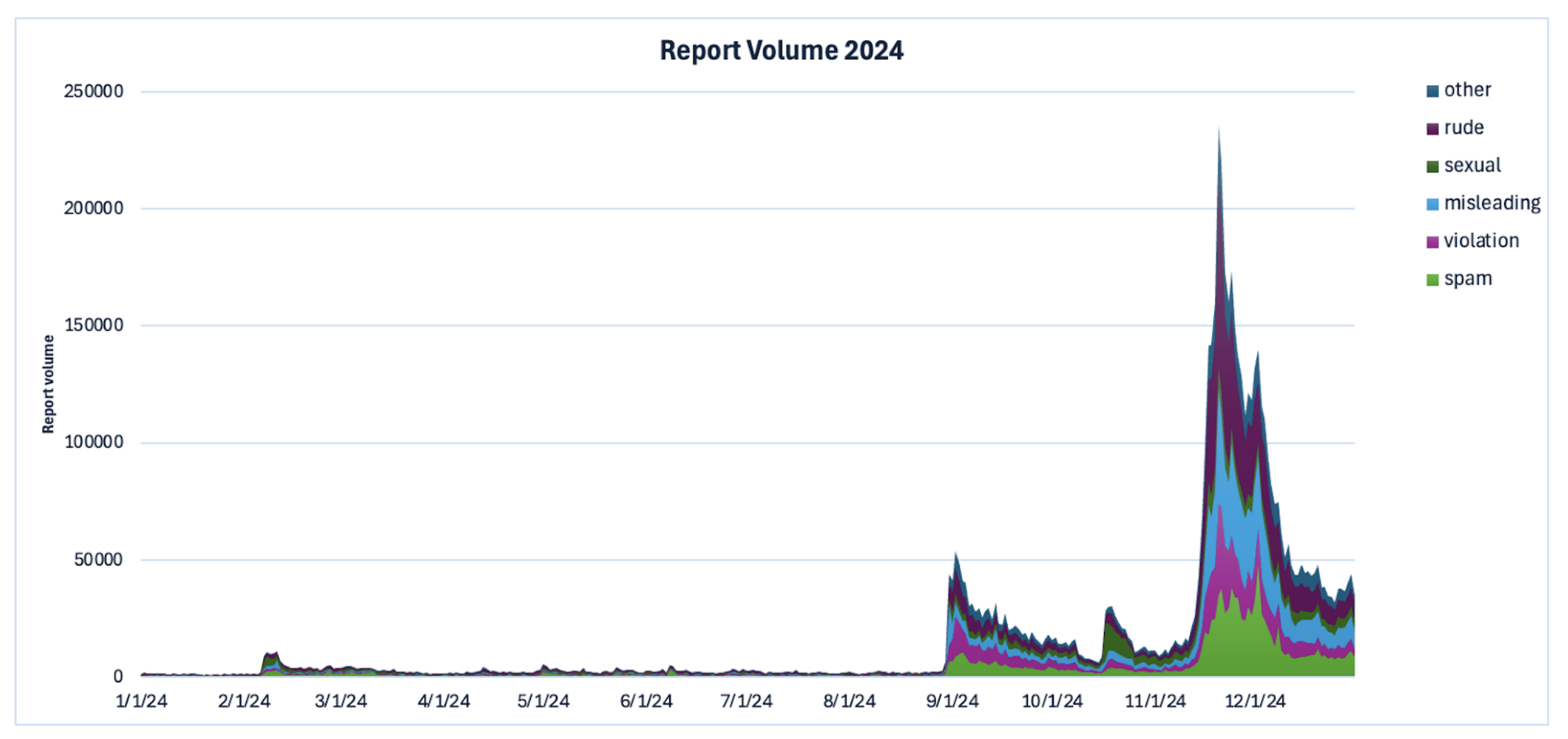
In late August, there was a large increase in user growth for Bluesky from Brazil, and we saw spikes of up to 50k reports per day. Prior to this, our moderation team handled most reports within 40 minutes. For the first time in 2024, we now had a backlog in moderation reports. To address this, we increased the size of our Portuguese-language moderation team, added constant moderation sweeps and automated tooling for high-risk areas such as child safety, and hired moderators through an external contracting vendor for the first time.
We already had automated spam detection in place, and after this wave of growth in Brazil, we began investing in automating more categories of reports so that our moderation team would be able to review suspicious or problematic content rapidly. In December, we were able to review our first wave of automated reports for content categories like impersonation. This dropped processing time for high-certainty accounts to within seconds of receiving a report, though it also caused some false positives. We’re now exploring the expansion of this tooling to other policy areas. Even while instituting automation tooling to reduce our response time, human moderators are still kept in the loop — all appeals and false positives are reviewed by human moderators.
Some more statistics: The proportion of users submitting reports held fairly stable from 2023 to 2024. In 2023, 5.6% of our active users1 created one or more reports. In 2024, 1.19M users made one or more reports, approximately 4.57% of our user base.
In 2023, 3.4% of our active users received one or more reports. In 2024, the number of users who received a report were 770K, comprising 2.97% of our user base.
The majority of reports were of individual posts, with a total of 3.5M reports. This was followed by account profiles at 47K reports, typically for a violative profile picture or banner photo. Lists received 45K reports. DMs received 17.7K reports. Significantly lower are feeds at 5.3K reports, and starter packs with 1.9K reports.
Our users report content for a variety of reasons, and these reports help guide our focus areas. Below is a summary of the reports we received, categorized by the reasons users selected. The categories vary slightly depending on whether a report is about an account or a specific post, but here’s the full breakdown:
- Anti-social Behavior: Reports of harassment, trolling, or intolerance – 1.75M
- Misleading Content: Includes impersonation, misinformation, or false claims about identity or affiliations – 1.20M
- Spam: Excessive mentions, replies, or repetitive content – 1.40M
- Unwanted Sexual Content: Nudity or adult content not properly labeled – 630K
- Illegal or Urgent Issues: Clear violations of the law or our terms of service – 933K
- Other: Issues that don’t fit into the above categories – 726K
These insights highlight areas where we need to focus more attention as we prioritize improvements in 2025.
Labels
In 2024, Bluesky applied 5.5M labels, which includes individual post labels and account-level labels. To give an idea of volumes, in Nov 2024, 2.5M videos were posted on Bluesky2, along 36.14M images. This comes primarily from automation where every image as well as frames from each video is sent to a provider for assessment, they return verdicts that match to our specific labels, and those are the ones you see from Bluesky Moderation. None of the images or videos are retained by the vendor or used for training generative AI systems. In June 2024, we analyzed the effectiveness of this system and concluded that the overall system was 99.90% accurate (i.e. it labeled the right things with the right labels). Human moderators review all appeals on labels, but due to backlogs, there are currently delays.
The top human-applied labels were:
- Sexual-figurative3 - 55,422
- Rude - 22,412
- Spam - 13,201
- Intolerant - 11,341
- Threat - 3,046
Appeals
In 2024, 93,076 users submitted at least one appeal in the app, for a total of 205K individual appeals. For most cases, the appeal was due to disagreement with label verdicts.
We currently handle user appeals for taken-down accounts via our moderation email inbox.
In 2025, we will transition to responding to moderation reports directly within the Bluesky app, which will streamline user communication. For example, we’ll be able to report back to users what action was taken on their reports. Additionally, in a future iteration, it will be possible for users to appeal account takedowns directly within the Bluesky app instead of having to send us an email.
Takedowns
In 2024, Bluesky moderators took down 66,308 accounts, and automated tooling took down 35,842 accounts for reasons such as spam and bot networks. Mods took down 6,334 records (posts, lists, feed etc.) while automated systems removed 282.
This month (January 2025), we’ve already built in policy reasons to Ozone, our open-source moderation tool. This will give us more granular data on the takedown rationale moving forward.
Legal Requests
In 2024, we received 238 requests from law enforcement, governments, legal firms, responded to 182, and complied with 146. The majority of requests came from German, U.S., Brazilian, and Japanese law enforcement.
In the chart below, User Data Requests are requests for user data; Data Preservation Requests are requests for Bluesky to store user data pending legal authorization to transfer the data; Emergency Data Requests are extreme cases where there’s a threat to life (i.e., someone actively discussing their own suicide with a time and date given for an attempt); Takedown Requests are requests for content removal; Subpoena Requests are largely user data requests, but if Bluesky fails to provide the data in a timely manner, it has to physically show up in court to defend not providing the data.
Type of Request | Requests | Responded |
User Data Request | 111 | 87 |
Data Preservation Request | 8 | 8 |
Emergency Data Request | 13 | 12 |
Takedown Request | 45 | 22 |
Inquiry | 44 | 36 |
Subpoena | 17 | 17 |
Totals | 238 | 182 |
The demand for legal requests peaked between Sept and Dec 2024.
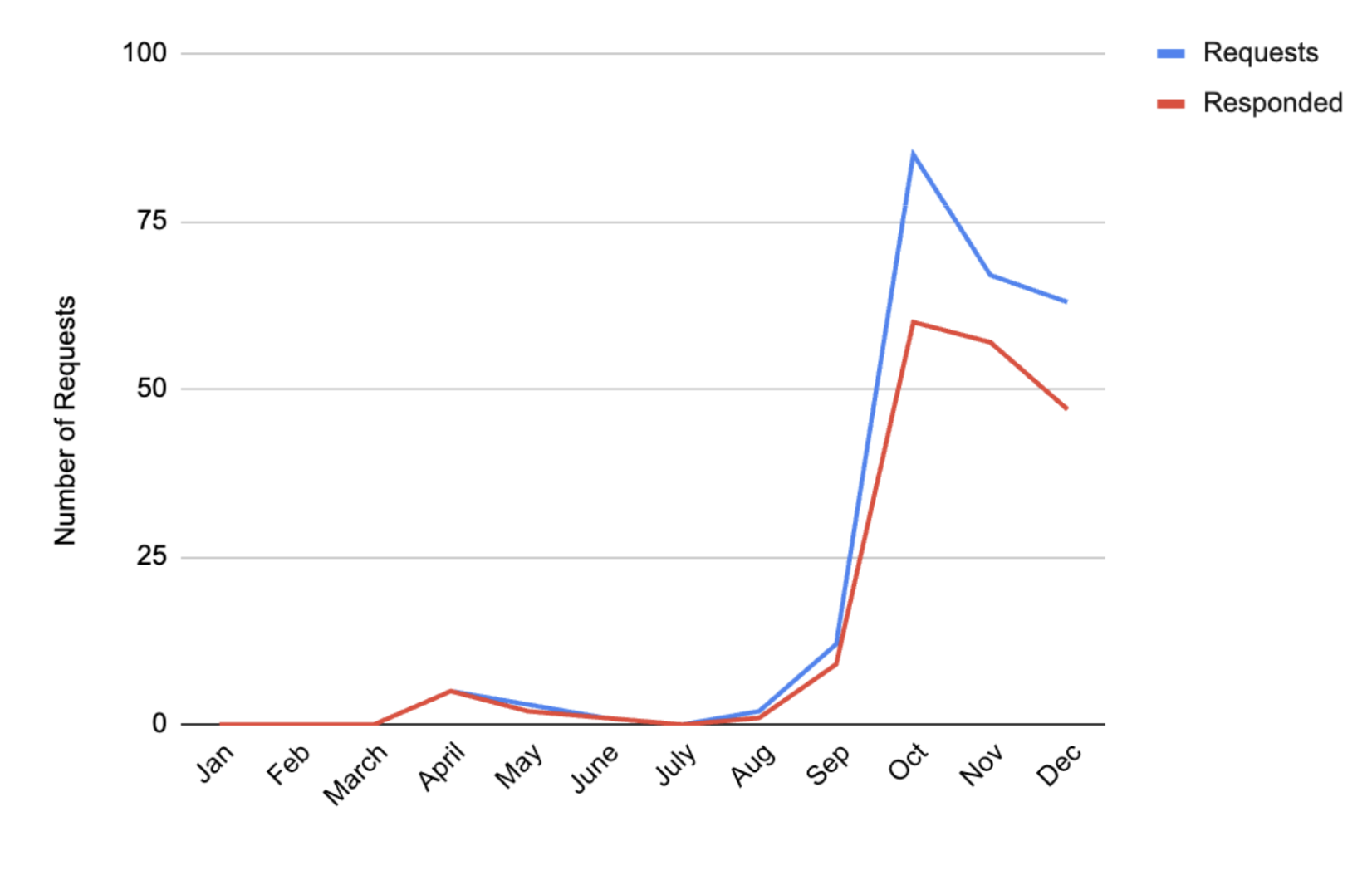
Copyright / Trademark
In 2024, we received a total of 937 copyright and trademark cases. There were four confirmed copyright cases in the entire first half of 2024, and this number increased to 160 in September. The vast majority of cases occurred between September to December.
We published a copyright form in late 2024, which provided more structure to our report responses. The influx of users from Brazil meant that professional copyright companies began scraping Bluesky data and sending us copyright claims. With the move to a structured copyright form, we expect to have more granular data in 2025.
Child Safety
We subscribe to a number of hashes (digital fingerprints) that match known cases of Child Sexual Abuse Material (CSAM). When an image or video is uploaded to Bluesky and matches one of these hashes, it is immediately removed from the site and our infrastructure without the need for a human to view the content.
In 2024, Bluesky submitted 1,154 reports for confirmed CSAM to the National Centre for Missing and Exploited Children (NCMEC). Reports consist of the account details, along with manually reviewed media by one of our specialized child safety moderators. Each report can involve many pieces of media, though most reports involve under five pieces of media.
CSAM is a serious issue on any social network. With surges in user growth also came increased complexity in child safety. Cases included accounts attempting to sell CSAM by linking off-platform, potentially underage users trying to sell explicit imagery, and pedophiles attempting to share encrypted chat links. In these cases, we rapidly updated our internal guidance to our moderation team to ensure prompt response times in taking down this activity.
To read more about how Bluesky handles child safety, you can find a co-published blog post on Thorn’s website.
Footnotes
-
users that have an account that hasn’t been suspended or deleted ↩
-
Not inclusive of GIFs through Tenor ↩
-
We haven’t managed to accurately separate figurative images from the rest in terms of our automated labelling as yet, so these are usually users who are appealing the automation applied labels on their art ↩